Human populations in the world’s mountains: Spatio-temporal patterns and potential controls
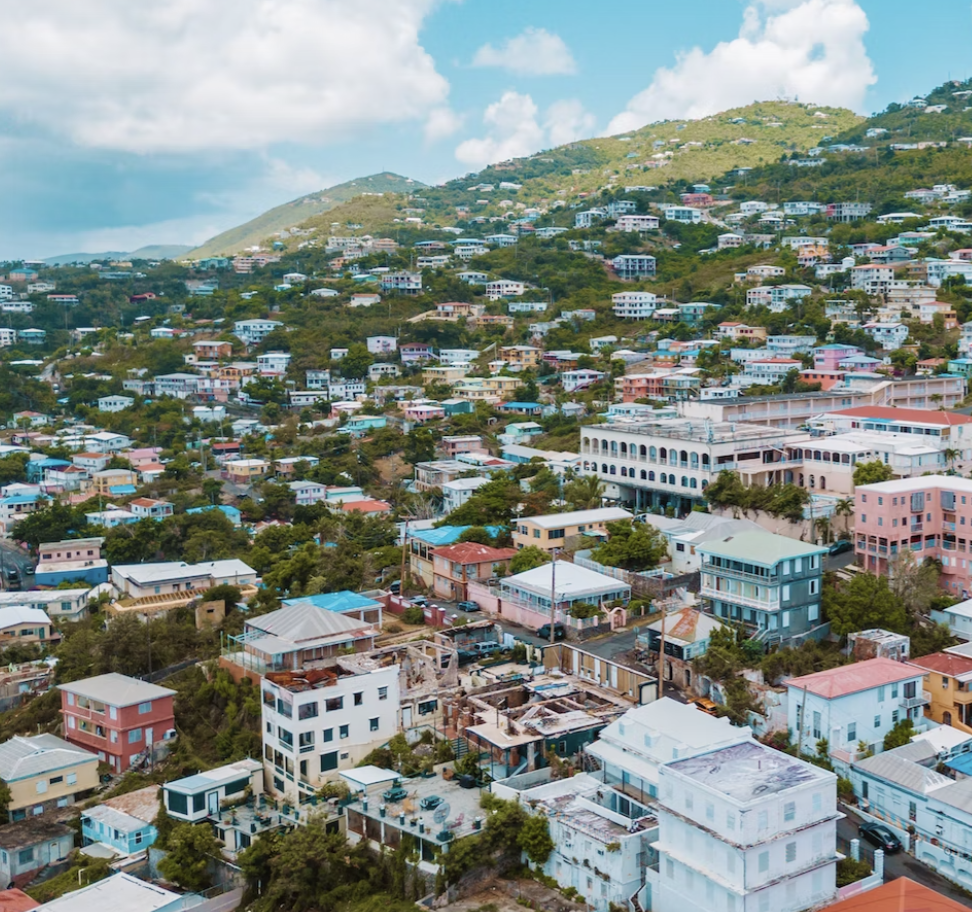
Introduction
Changing climate and human demographics in the world’s mountains will have increasingly profound environmental and societal consequences across all elevations. Quantifying current human populations in and near mountains is crucial to ensure that any interventions in these complex social-ecological systems are appropriately resourced, and that valuable ecosystems are effectively protected. However, comprehensive and reproducible analyses on this subject are lacking.
This study develops and implements an open workflow to quantify the sensitivity of mountain population estimates over recent decades, both globally and for several sets of relevant reporting regions, to alternative input dataset combinations. Relationships between mean population density and several potential environmental covariates are also explored across elevational bands within individual mountain regions (i.e. “sub-mountain range scale”).
This article is an abridged version of the original text “Human populations in the world’s mountains: Spatio-temporal patterns and potential controls” published in PLoS ONE, 17(7), under the CC0 1.0 license in July 2022. Please note that references have been removed.For more detail, full references, and to quote text please use the paper available for download on the right.
Materials and Methods
Data selection and preparation:
The method employed entirely open-source datasets and software tools. The datasets used can be grouped into the following categories:
- Mountain delineations
- Population grids
- Aggregation/reporting polygons
- Urban area extents, and
- Other potential population covariate datasets (e.g. topographic, climatic, and protected-area extent layers).
Processing workflow overview:
Although the workflow is conceptually rather simple, its implementation for such a large combination of (large) databases was not trivial. Given the size of certain input datasets and the extensive and efficient geospatial functionality required, PostGIS—an extension that adds spatial functionality to the widely used, open source PostgreSQL object-relational database system—was used.
The workflow can be separated into three somewhat distinct components.
- Part A consists of a comprehensive analysis to derive various area, population, and population density estimates for all considered combinations of mountain delineations, population grids, and years. Results are reported both at the global level and against the five different regional aggregation/reporting polygon datasets.
- Part B is concerned with the quantification of urban mountain populations, and involved only a subset of the population grids used in Part A. For internal consistency, results were not generated for combinations of urban extents and population grids that did not correspond to exactly the same year.
- Part C sought to explore the patterns and dependencies between mountain population density / urbanization estimates and several potential environmental covariates summarised across three elevation bands within each mountain region, which we refer to as sub-mountain range scale. To investigate the extent to which projected future mountain population metrics might be related to topographic and (present day) climatic and protected-area variables, additional correlation matrices (both the simplified and more detailed version) were generated by substituting population metrics derived from the five (global) Shared Socioeconomic Pathways (SSPs) for the years 2050 and 2080.
To explore the method in more detail, please refer to pages 4-7 in the full paper (available to download on the right-hand side).
Key Findings
The study’s main findings are that:
- Variability in mountain population estimates is dominated by the choice of mountain delineation. Population dataset choice is not negligible, however, especially for smaller spatial analysis units; for instance, substituting GHS-POP for WorldPop causes a change of up to 39.9% in the estimated global urban mountain population, all else being equal.
- In many mountain regions, population increases over recent decades have been associated with strong urbanization in both extent and population, although population and urbanization trends are disconnected in some regions (see Figs. 2 and 3 below).
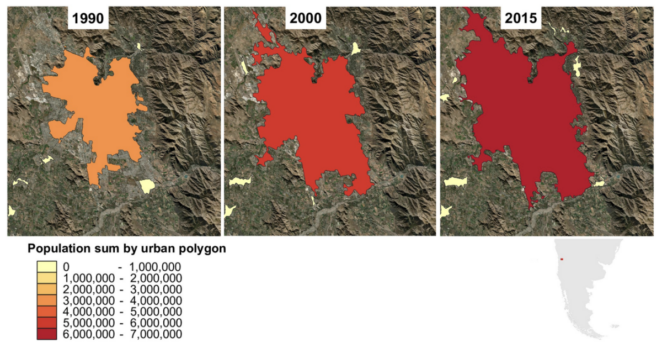
- In parts of Africa especially, mean population densities in mountainous regions are notably higher than densities more generally. This suggests that, broadly speaking, mountains provide important “refugia” for human populations in certain dry and/or hot climates (refer to Fig. 4 in the full article).
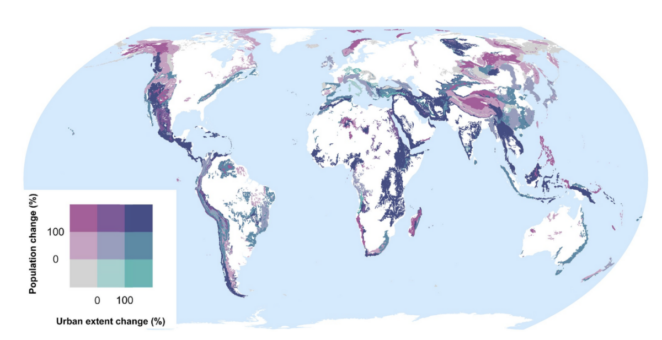
- At sub-mountain range scale (i.e. within individual mountain regions), moderate and high mountain population densities are found to occur under a relatively wide range of climatological and topographic conditions (see Fig. 8 below). That said, some evidence of climatic controls, especially measures of temperature, exists. It certainly appears that climatic variables may generally exert a stronger influence on mountain densities than topographic variables, at least at the spatial scales considered.
- Moreover, in many instances, these dependencies appear to have strengthened through time as population growth has preferentially occurred in regions with more favorable conditions. Overall, these findings align with those of previous, global studies that have analysed the influence of more specific population covariates for both pre- and post-agricultural/industrial societies.
- Correlations between population metrics and potential covariates evident over the historical period are not consistently maintained when future global population projections are substituted; this could indicate either that these influences break down under future conditions, or else that future population projections somewhat overlook the specific factors that affect mountain social-economic systems and populations. Further work in this area is required, however.
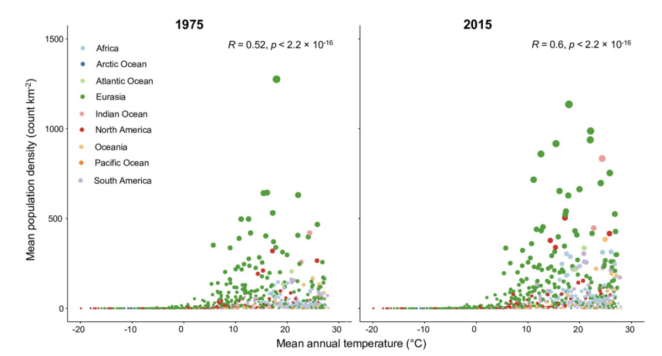
Refer to pages 7-17 to learn more about the study’s results and discussion, and to explore the figures in more depth.
Conclusions and Limitations
Limitations and future study:
- Whilst individual mountains can be easily identified and mapped as discrete landform entities, across larger areas the distribution of mountains is often more scattered and/or mountains are separated by inter-montane valleys of variable widths. All established delineation approaches identify such areas as mountain regions despite them containing non-mountain landforms like hills, valleys, and forelands. This is important because it is this non-mountainous terrain that often becomes urbanized. The unavoidable ambiguity explains our insistence that the expression “mountain (urban) population” should actually be taken to mean “(urban) populations living in and near mountainous regions”.
- Notwithstanding the above, by providing estimates mainly on the populations living “in” (or very near) mountains themselves, the study did not quantify the populations in connected lowland regions that currently benefit from mountain ecosystem services, and who may therefore also be exposed to any adverse impacts of mountain system change.
- The gridded population datasets used are also subject to uncertainty, much of it related to the methods by which census data are disaggregated.
- Numerous additional factors that could strongly influence mountain populations were neglected to keep the scope manageable. In particular, looking ahead, it could prove fruitful to include socio-economic and demographic variables like birth rates and age distributions, data on healthcare and education, transport and digital connectivity, economic conditions for agriculture and other activities, levels of governance and outside investment, efficiency of responses to past natural hazard events, and the perception of efforts to mitigate the impacts of future events.
- The introduction of future climate projections to address possible dependencies between projected future climate and population patterns in mountains fell beyond our scope.
Explore the study limitations and areas for future outlook in more detail on pages 17-20.
Conclusion:
In summary, this study provides a sound and objective basis for various important subsequent applications involving people and mountains across traditional disciplinary boundaries and spatial scales. The authors recommended that users of mountain population estimates engage more closely with the various underlying methods and data choices to develop a fuller appreciation of how conclusions and ultimately important decisions are made. There is great potential for the datasets generated by our fairly comprehensive, comparative analysis to be further exploited and/or developed. Finally, the scripts provided may also prove useful for spatial analyses in other disciplines involving similarly large and varied spatial datasets.
Suggested citation:
Thornton, J. M., Snethlage, M.A., Sayre, R., Urbach, D.R., Viviroli, D., Ehrlich, D., Muccione, V., Wester, P., Insarov, G. and Adler, C. (2022) Human populations in the world’s mountains: Spatio-temporal patterns and potential controls. PLoS ONE 17(7): e0271466. https://doi.org/10.1371/journal.pone.0271466
Related resources
- Mountain observations: Monitoring, data, and information for science, policy, and society
- Leave No Mountain Behind: Disaster Risk Reduction for All
- Mountain Observatories: Status and Prospects for Enhancing and Connecting a Global Community
- COP27 - Cascading impacts: how can we adapt and reduce risk in the mountains and downstream?
(0) Comments
There is no content